In 2022 I wrote about pioneering work into deep learning and AI-enhanced imaging to deliver superhuman surgical advances to the medical industry. In the blog I mentioned that we were taking a multi-frame approach to improve image accuracy – great in research but too slow for use in surgery. A couple of years on, I’m thrilled to share news of significant progress. Our patented Omniscia™ imaging platform now sets the standard for state-of-the-art, real-time AI based video super resolution.
It uses a custom multi-frame approach and a deep understanding of AI that leverages NVIDIA’s Holoscan platform to deliver sharper, higher resolution, higher contrast images with lower latency than any other currently published technique.
How important is the breakthrough? Well, I’d say it represents the possibility of a significant shift away from traditional computer vision towards AI based systems. Our Omniscia demonstration at the Hamlyn Symposium for Medical Robotics 2024, a conference focused on advanced research in the field, surprised many delegates and exceeded the expectations of surgeons and researchers by seemingly achieving impossible image enhancement over the state of the art. We had a non-stop interest for the two days we were there.
One eminent surgeon was understandably sceptical. Our demo showcases the performance enhancement in a conventional laparoscopic surgical scene by allowing visitors to use real tools in the scene to get a feel for the low latency. He could see it was good but also appreciated that AI image enhancement systems tend to fall over when presented with something they have not been trained on. Our expert duly introduced his glass of white wine into to the surgical scene, and was astounded by the picture-perfect, super-resolution result, commenting “How the [heck] does that work!?”
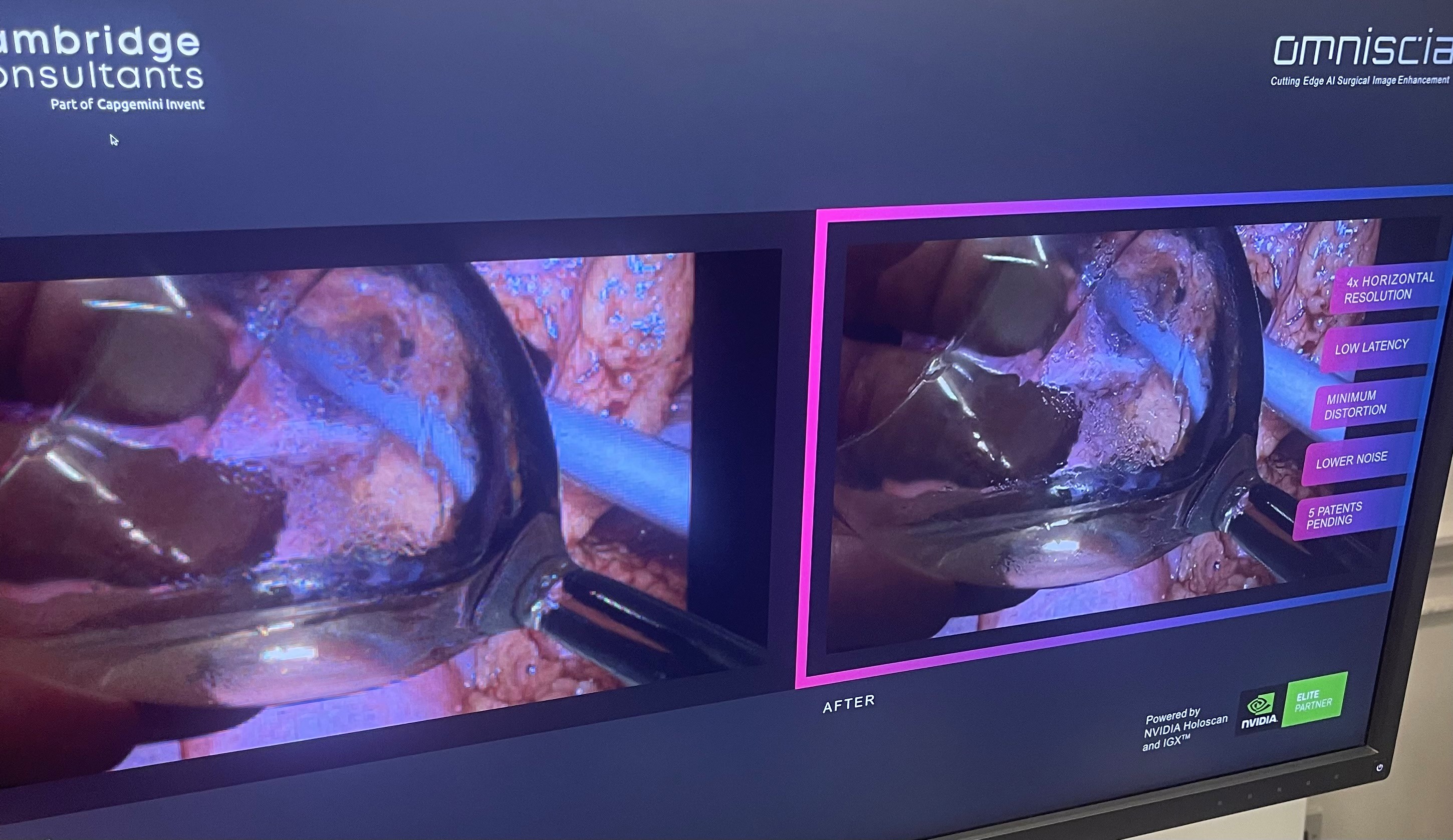
The Omniscia superresolution algorithm uses only in-scene information to generate high resolution outputs. Unlike pure Gen-AI approaches, it’s robust to objects that it’s never seen before, such as this sceptical surgeon’s wineglass.
AI-based digital surgery imaging
To explain why he was so surprised and put the significance of our progress into perspective, let me explore a bit more background. Broadly, digital surgery stands to benefit greatly from novel AI-based imaging approaches that increase resolution and make noisy visual data interpretable. But they haven’t been adopted because purely generative AI techniques risk hallucinating information (see my previous post for details). In removing the noise, they can also inadvertently smooth over features of interest – particularly where these are rare, like polyps or critical structures – creating unseen risks for the surgeons.
This is, of course, a highly pertinent challenge for surgery – which demands accuracy and snappy, low-latency performance. When surgeons are stitching or cutting tissue which is moving within the body, they need to be able to trust what they are seeing, adapt and act in real time.
Please be aware the following video contains surgery footage:
Our innovation in AI algorithms
In simple terms, our AI system works differently. By tracking the motion of objects in the scene, we are able to sum multiple frames together creating higher quality information per frame. This is a technique used in astrophotography, but we’re doing it in 12ms not three days. Our unique way of using the extra bandwidth offered by using multiple video frames to take information from the time domain and placing it in the visual domain allows us to address the most common challenges in image signal processing. Our imaging stack includes novel super-resolution, adaptive contrast and de-noising algorithms which allows crisper images from a wider range of camera hardware than ever before.
Our key points of progress over the previous state-of-the-art are:
- Adaptive contrast with 100x reduction in noise.
- 4x increase in resolution enhancement.
- 12ms latency (faster than a single frame at 60fps).
- 20x faster development time thanks to imaging pipeline entirely defined in software.
These achievements are protected by several patents we’ve taken out around key breakthroughs. They include optimising performance of algorithms against human perception; achieving real-time super resolution that is robust to real world conditions; removing smoke and distortion; and improving contrast.
Shifting to an AI based pipeline does require a change in thinking, but the advantages quickly outweigh the drawbacks as NVIDIA’s Holoscan platform and CUDA make available a wide range of library functions meaning that we only had to focus on the novel aspects to achieve real world beating performance.
High-criticality AI support tool
The innovation is very much in the CC tradition of developing AI systems that enhance and augment the human imperative rather than replacing it. What we’re aiming for is a user experience that is so snappy and seamless that the AI itself falls away into the background to become an intrinsic part of the workflow. Omniscia can be characterised as a high-criticality support tool that works in concert with an expert human user.
In these terms it has capabilities that are suitable for a wide range of industry sectors and applications, from RADAR to automotive scene reconstruction. We’ve got great plans for platform development in the coming months, and have already added real-time zero-shot tool and scene tracking allowing surgeons to fully quantify their workflow without tediously labelling thousands of examples.
Our advances in this field come as a result of combining expertise from a multidisciplinary team of scientists, engineers and designers who remain committed to a systems-thinking approach to create surgical tools that enhance clinical workflows. Do please reach out if you’d like to discuss any aspect of our work in more detail. We’re ready to discuss the merits of Omniscia with potential clients who want to develop their own applications – and accelerate their R&D programmes by 20x.