Client story
In order to test our capabilities at the cutting edge of artificial intelligence (AI), we set ourselves the challenge of creating a machine learning system that could address the infinitely complex world of music.
The result is The Aficionado – the first and only machine learning system that can accurately identify the musical style of live piano music.
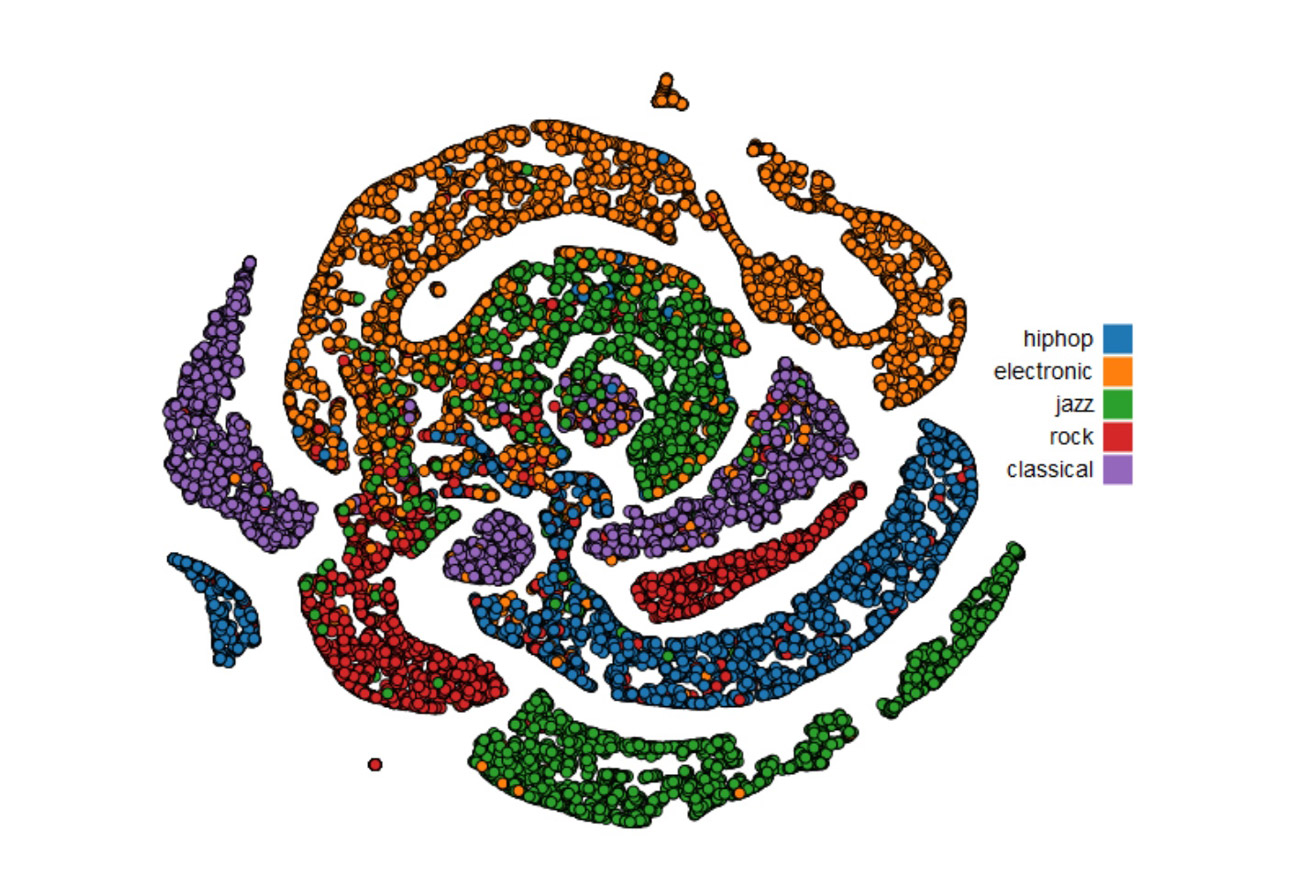
Human-like judgement
Using deep learning technology cultivated in our Digital Greenhouse, The Aficionado quickly and accurately classifies solo piano music in real time.
The AI consistently and overwhelmingly outperforms classical hand-written software algorithms – both in terms of speed and accuracy – showing uncannily human-like judgement.
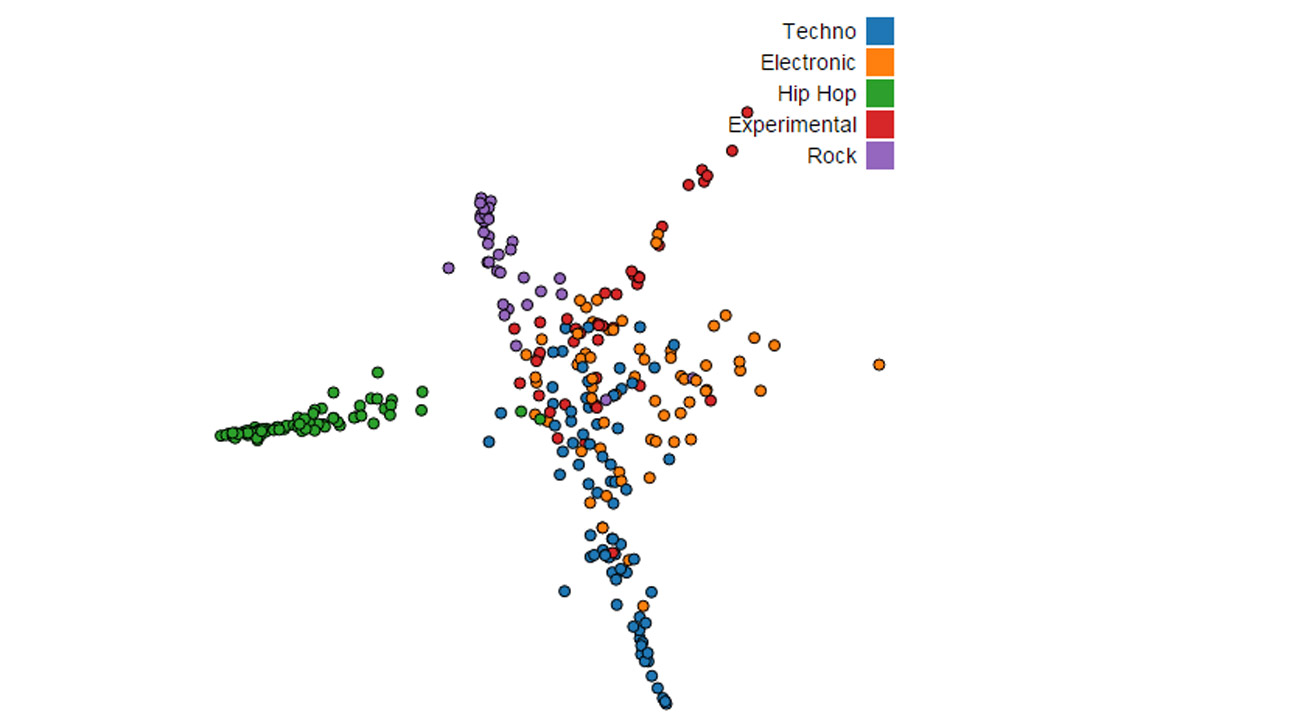
Unprecedented automation potential
Though its focus is music, The Aficionado illustrates the ability of machine learning to identify and recognise patterns in complex data.
From detection of faults in an industrial system to rapid assessment of patient health from sensor waveforms, the potential applications – and scope for commercial advantage – are endless.
“We’re working at the cutting edge of machine learning research and development, developing systems that can apply complex algorithms to big data and ‘learn’ autonomously – without explicit programming.”
Our involvement
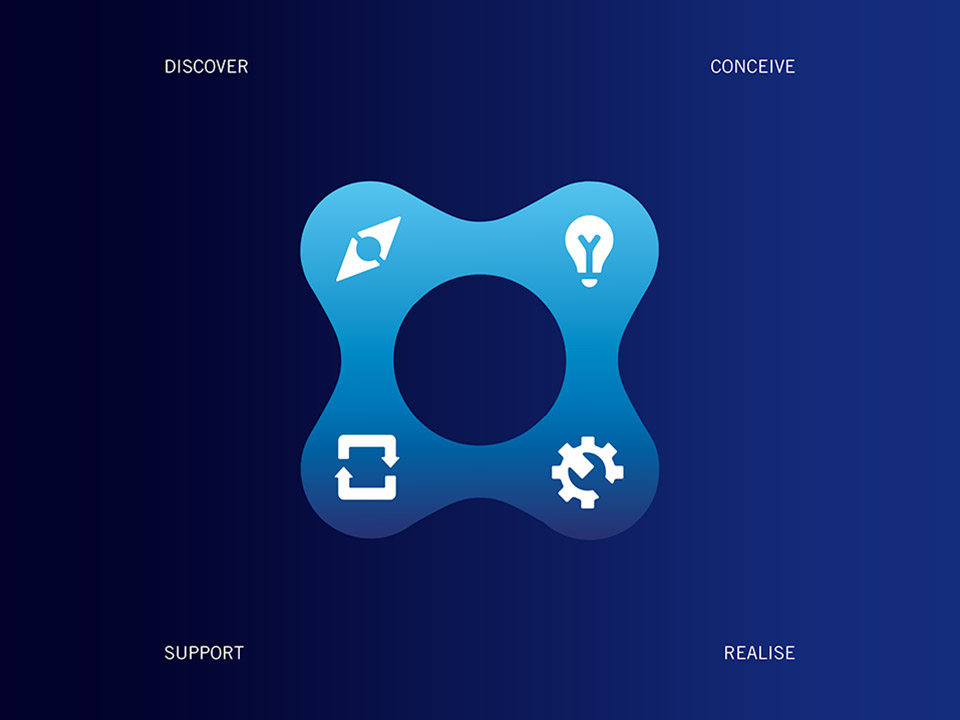
As an internal experiment that grew organically out our Digital Greenhouse, our approach to The Aficionado was unlike any client project we have ever undertaken.
We picked a problem that was deemed almost impossible and continuously developed, tested, learned and re-developed our machine learning system throughout the project.
The challenge
We set out to build a machine learning system that could correctly classify different musical styles in real time – something that had never been done before – from concept to completion in just three months.
We didn’t want the system to simply learn the music – we wanted it to actually understand the music.
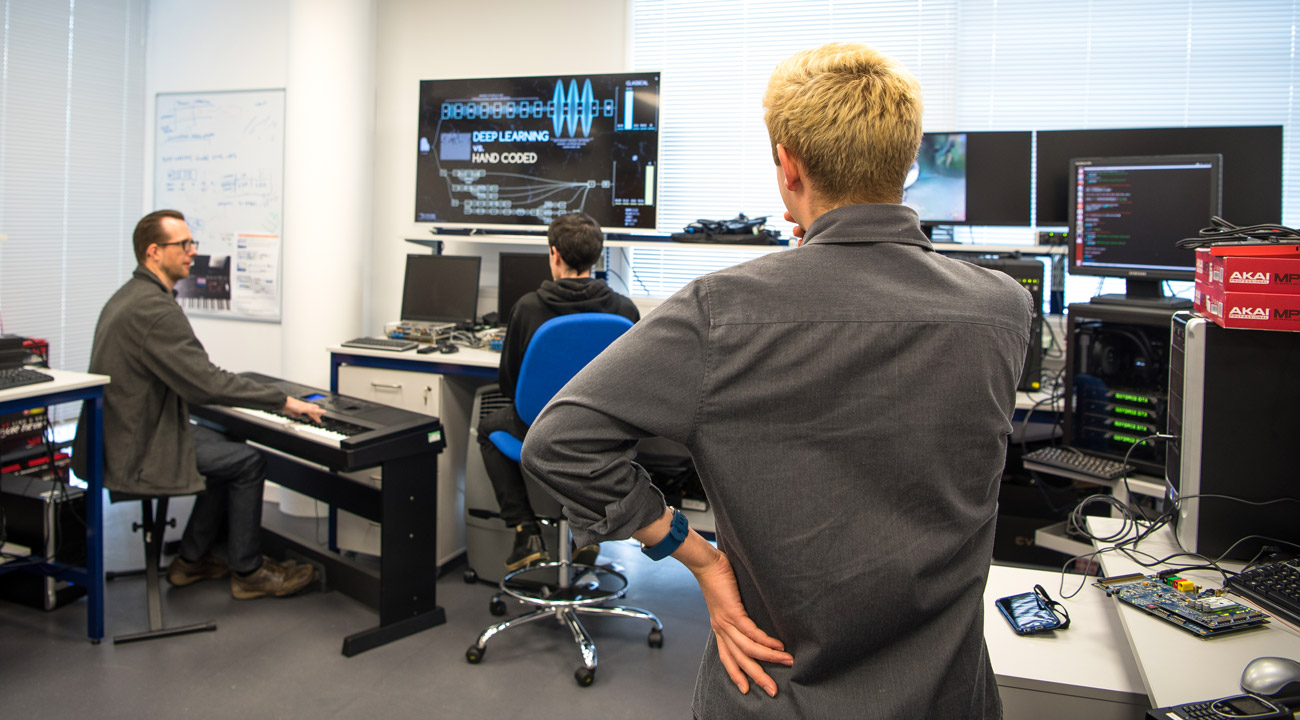
Creating the Digital Greenhouse
The Digital Greenhouse is both a physical facility and an experimental approach to developing and perfecting machine learning.
The ‘greenhouse’ analogy is an apt one, as it is here that our technologists breed and test new models of machine learning, using state-of-the-art computing resources to assess new algorithms against calibrated challenges. The more new strains we grow, the better we understand where the richest pickings are to be found.
Real-time analysis
Performing machine learning in real time is inherently complex. We started by tackling distinctly different genres of music, such as hip-hop, classical and rock. This gave us an early indication of the challenges we would face with solo piano music and helped us home in on the best deep learning techniques.
We quickly discovered that, in order to achieve real-time analysis, we could not rely on off-the-shelf toolboxes. Instead, we needed to develop a fully bespoke deep learning system.
We used our own in-house GPU-powered data farm – providing far superior speed compared with cloud services – together with a dedicated machine for processing the piano audio signal and carefully chosen deep learning architecture to deliver near-instant results.
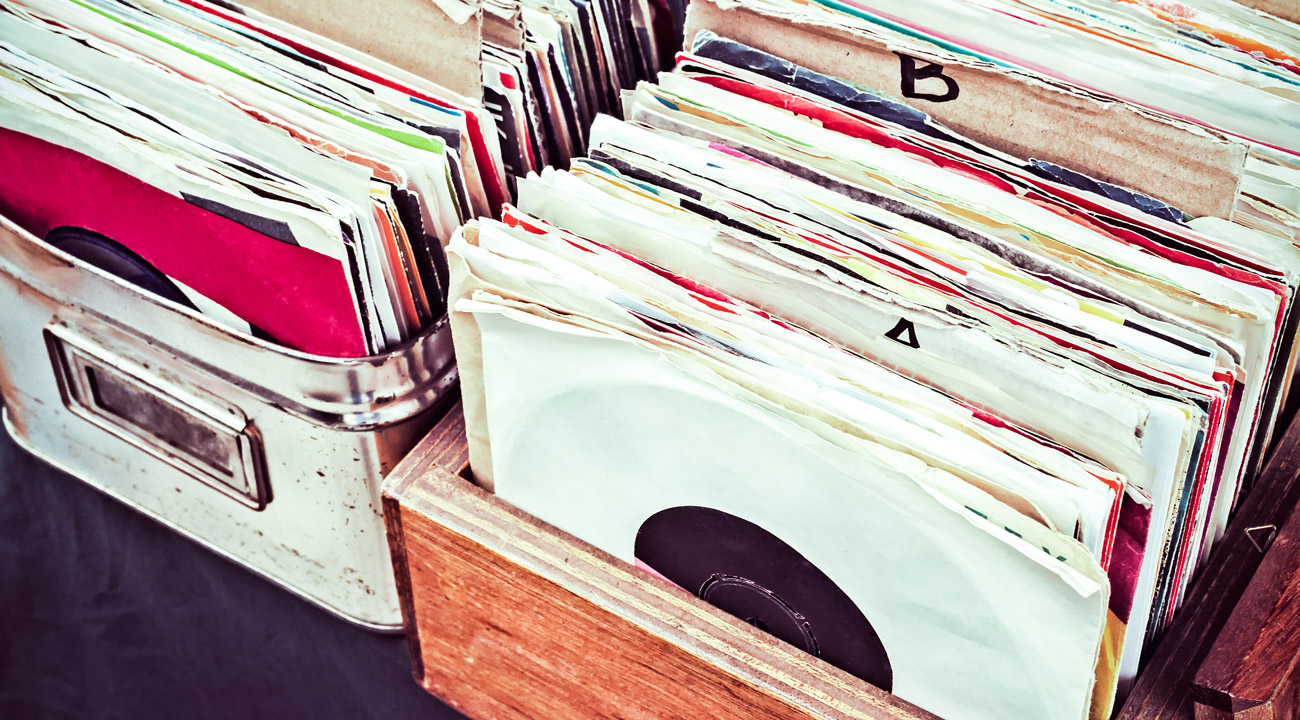
Dealing with noisy data
Practical machine learning using real-world data is an entirely different proposition to using generic datasets.
We intentionally fed The Aficionado messy data – such as practice sessions featuring barking dogs, crying babies and other background noise, and synthesised data by subtlety changing the sound, tempo and rhythm of the playing – to increase the quantity and complexity of examples it learned from.
We then applied expert data science to teach The Aficionado to generalise, ensuring consistent genre outcomes from any pianist on any piano.
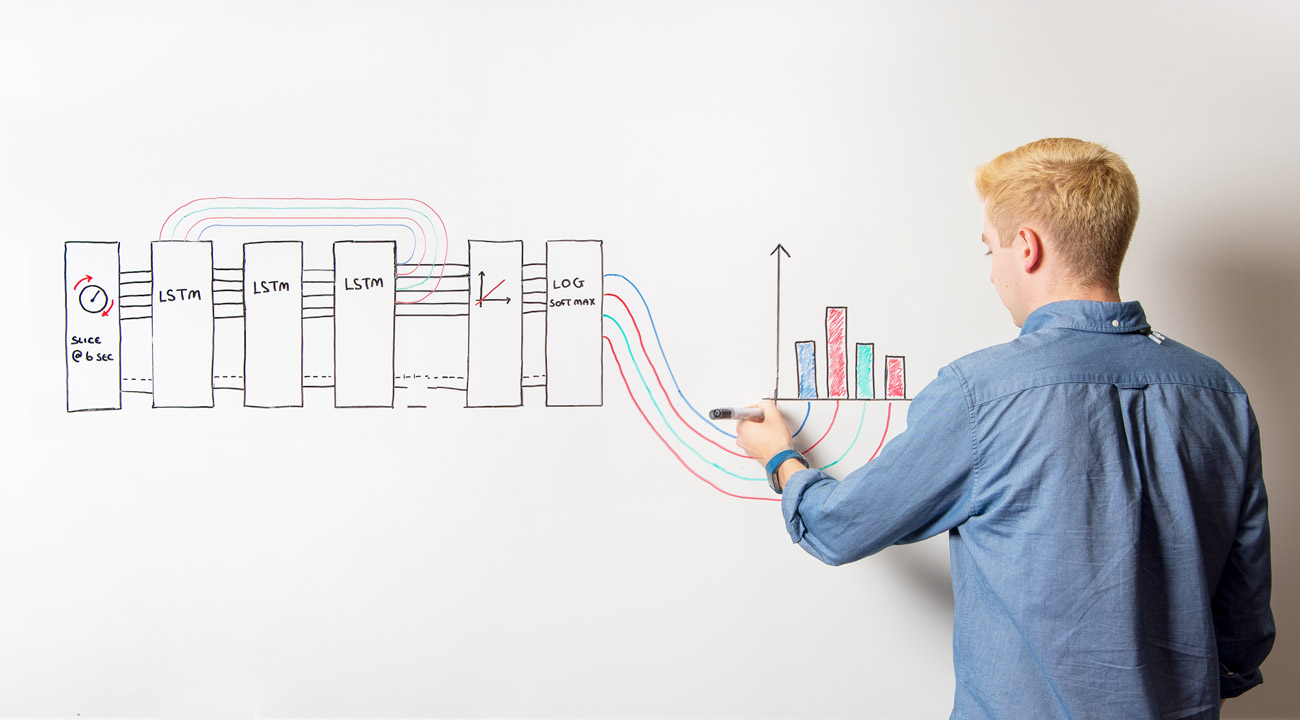
AI vs hand-coded software
An important part of The Aficionado project was to compare how the AI fared against conventional hand-coded software. Could the machine find a better way of telling one genre from another than classic code?
In practice, The Aficionado conclusively outperformed the software written by our engineers, proving more accurate and more decisive in classifying the genre of the music being played. To demonstrate this, we created a graphical interface showing the real-time output of the two approaches side-by-side.
The team
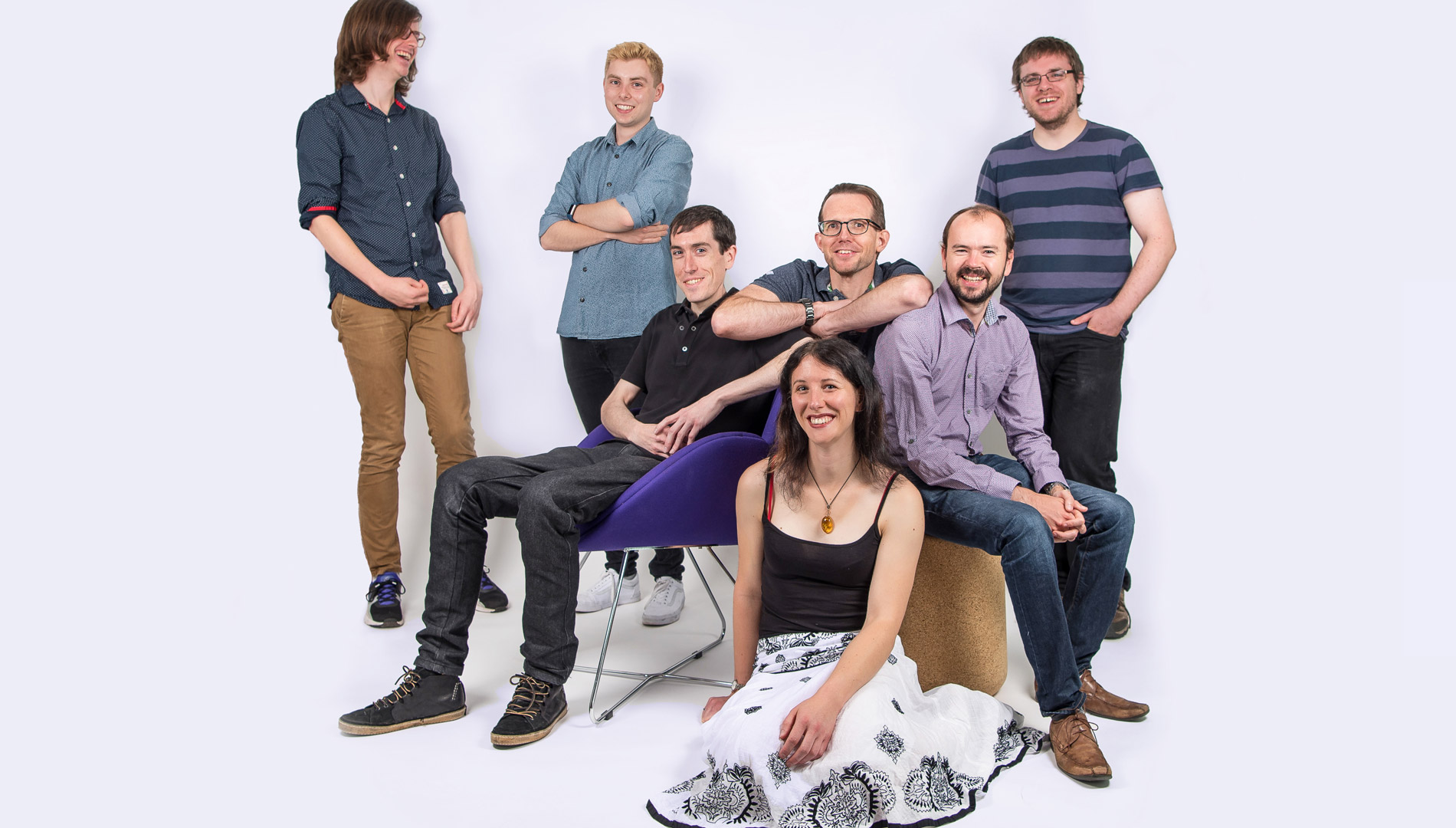